Gen AI: A game changer for biopharma operations
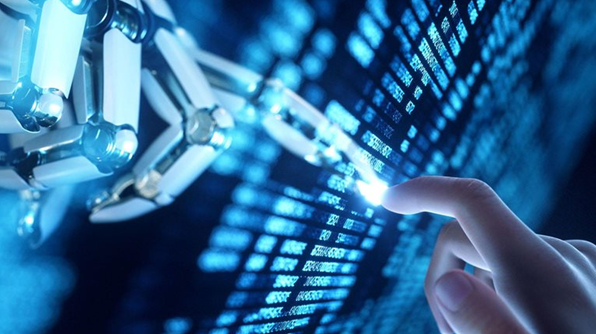
Gen AI has become a hot topic among leaders at most big companies, more than two-thirds of which intend to increase their investments in the technology over the next three years.1 McKinsey has estimated the opportunity for gen AI in biopharmaceutical operations at $4 billion to $7 billion annually through workload and cost reductions, productivity gains, improvements to equipment effectiveness, and quality enhancements. Many biopharma organizations now have sophisticated operations and are moving to use gen AI to take advantage of their data repositories. However, only a few organizations have started to realize value from it. This raises a question: Are organizations deploying gen AI in the places with the highest potential benefits?
Over the past five years, biopharma has adopted various digital and analytics solutions, including in silico models, process optimizers, and lab and manufacturing automation.2 This article explores how gen AI, which differs from traditional AI and other analytics, can help biopharma solve industry-specific challenges.
There are at least a dozen proven and potential gen AI use cases along the biopharma operations value chain. They fall into three main categories:
- entry-level use cases that biopharma organizations and vendors are already deploying using off-the-shelf products
- novel use cases that incorporate product, technology, organization, and domain-specific intelligence and require custom, in-house development
- frontier use cases that typically require fast processing of large amounts of real-time numerical data and affect elements of operations that require tight quality and regulatory oversight (Exhibit 1)
Vendors continually implement and improve entry-level use cases in their products. For instance, enterprise-resource-planning vendors are employing gen AI to improve such functions as demand forecasting, inventory management, and transportation planning. Many smaller companies are also developing gen-AI-supported point solutions for those entry-level use cases.
At times, companies need to customize the solution to fit their needs and workflows better, but they generally do so in partnership with the relevant vendors. So to take advantage of the existing technology, biopharma companies need only buy or upgrade software as it becomes available.
At the other end of the spectrum are frontier use cases, which are too risky today for most companies to want to explore. In this article, we focus on four novel use cases that require bespoke development but are within the current capabilities of gen AI. Agentic AI use cases, which incorporate a layer of autonomous execution, build upon other AI applications and fall into this category as well.